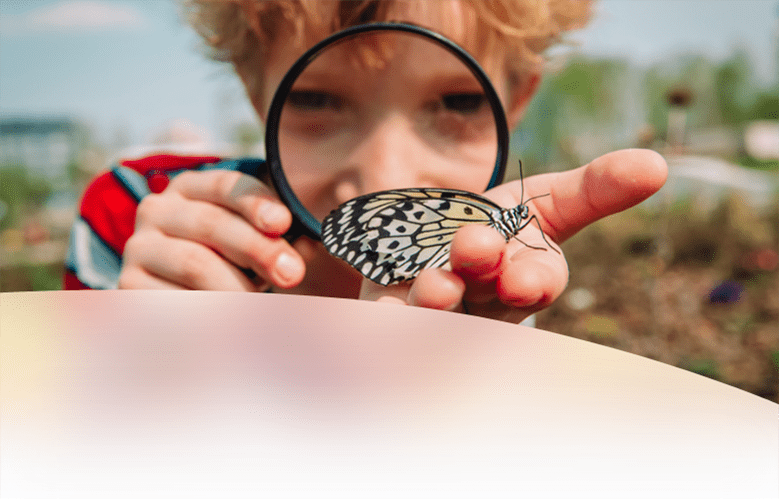
Line Optimization
Choice Based Conjoint
Solution
Strategy
Development
Landscape
You may be familiar with typical product-based conjoint, where the individual features of potential products are assessed to create the best overall product package. Line optimization conjoint modeling works similarly, but it takes things up a notch strategically; instead of revealing the optimal product from a set of variable attributes, it determines the optimal portfolio from among a set of variable products.
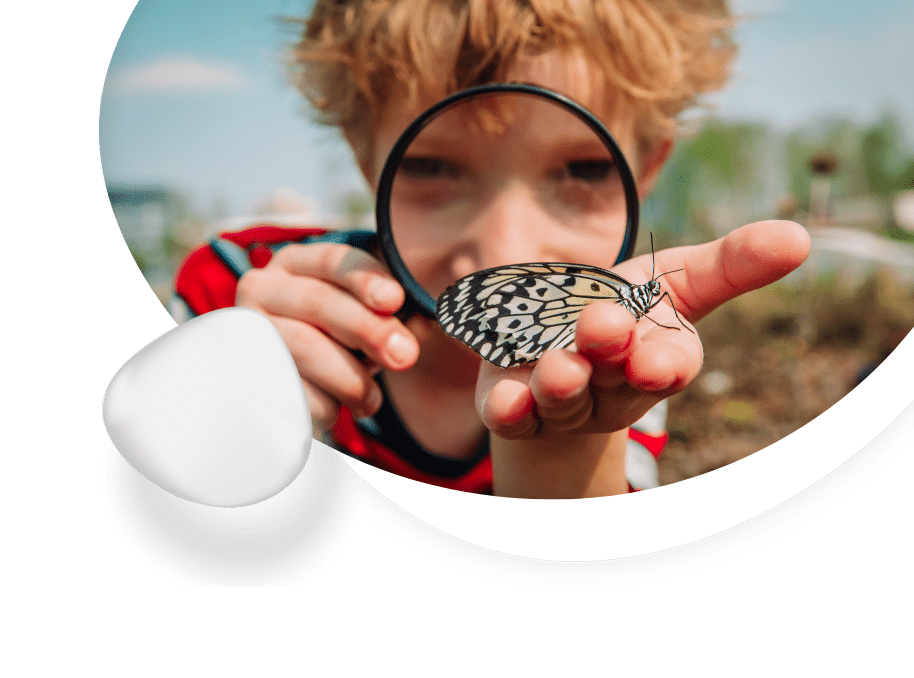
- Custom market simulator
This interactive analysis tool allows researchers and strategists to create and evaluate numerous scenarios from among a set of products and prices. This simulator reveals impacts of changes to individual items, sublines, and brands as a whole. It even includes filters so that analysis can be easily conducted among subgroups of interest. You’re also able to leverage the full analytical power of the Research Service team, who will use this tool to develop the answers to your research questions into a comprehensive report.
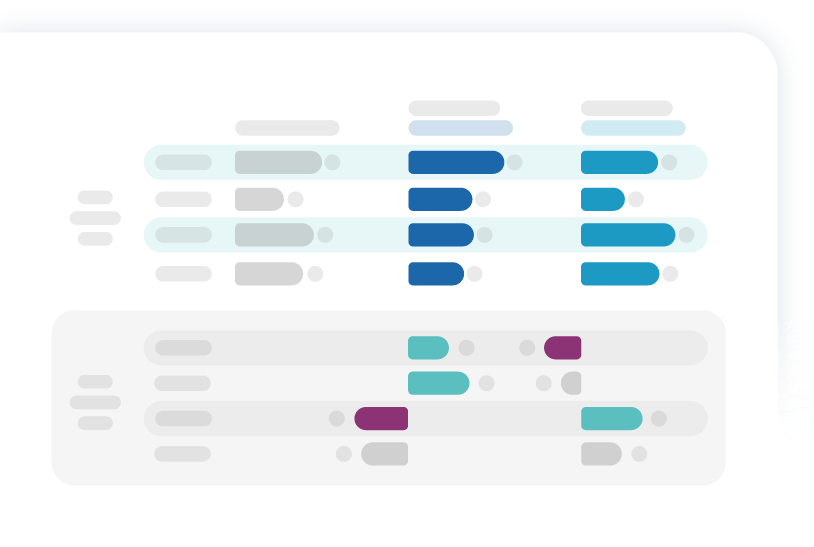
- Preference share
At the individual level, and aggregated by subline/brand/category - Revenue per 1000 consumers
Preference share factoring in tested price points - Preference likelihood
Value between 0 and 100 representing the likelihood of a particular option being selected
Portfolio analysis
Which existing products should we delist as we bring new products to market?
Will our new product(s) cannibalize our existing products?
Product development and pricing
Which new product/sublines should we introduce to optimize our total portfolio?
What pricing can these new products command?
Business insights
Will our new product(s) yield trade-in from top competitors?
Will our new product(s) yield trade-up from our value customers?
All potential products and prices are input into the conjoint test—these are the “raw materials” with which the predictive models will be built. After screening into the survey, the conjoint portion begins and the task is typically framed as a “shopping” activity which ensures the respondent is in the right headspace to make selections based on their preference.
Respondents then see a handful of conjoint-generated combinations of items and prices, and are asked to select their most likely “purchase.” They perform this task a number of times, each time with a new and varied set of products.
Behind the scenes, utility scores are calculated and conjoint algorithms are generated to feed the simulator with the information it needs to simulate the resulting share of preference across any potential product lineup.