Survey authoring tool
Build with ease and precision
Our agile survey builder is designed to satisfy your curiosity—no matter how simple or sophisticated that may be. Program, view, and edit even the most complex surveys on one page with a simple drag-and-drop interface—all with a growing toolbox of powerful research test automations.

One place for all of your research needs
Our agile survey editor puts a collection of quantitative and qualitative survey question types, advanced research tests, and powerful survey logic at your fingertips with an easy-to-use interface.
Collaboration
made simple
Working with a team? Share your survey and allow team members to comment, edit, and collaborate before fielding. Our question library lets you save frequently used survey questions, add them to any future survey, and share them for simplicity and consistency.
Get support
when you need it
We have a vast collection of videos, tutorials, and instructional documents to help inform you throughout every step of the process. If you need any other help, our client support team is always just a call or a click away!
Empower your curiosity
Our powerful automation solutions will help you find answers and advance your ideas without having to sink countless hours and resources into programming. We’ve carefully crafted a comprehensive index of question types, powerful features, and advanced research tests to address your research objectives—all complete with advanced survey logic and built-in best practices.
State-of-the-art
question types
Our agile survey tool lets you leverage powerful question types to get the answers you need. Upload images, randomize your questions and subquestions, and maintain complete control over your respondent experience. Click the question types below to watch a short video.

Turnkey research tests
Sometimes surveys need more than just questions. Sometimes they require advanced methodologies, sophisticated programming, and lots of number crunching. Our constantly growing collection of cutting-edge automated research tests help you get the answers you’re looking for—complete with automated analysis.
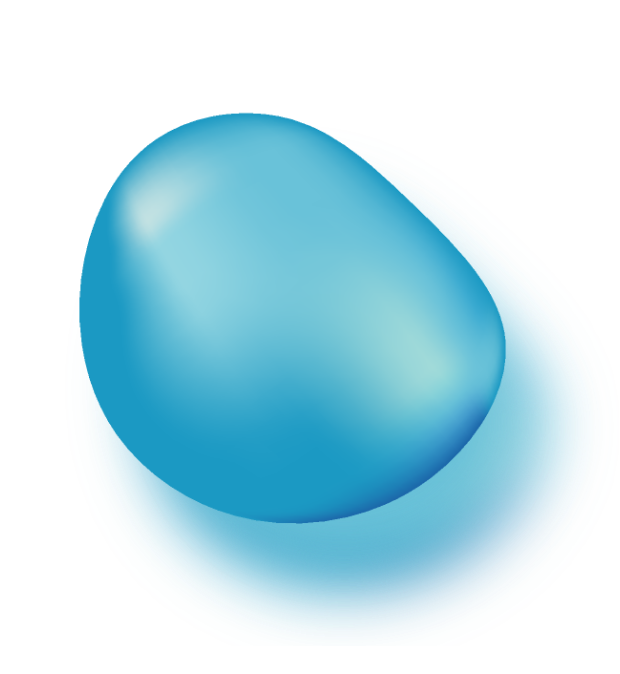
aytm survey logic
Our advanced logic empowers users to create a tailored survey experience, giving them direct control over the question and answer options shown to respondents. The result? A hyper-relevant survey experience designed to reduce respondent fatigue, mitigate order and exposure bias, and ensure that your insights are based on high-quality data.
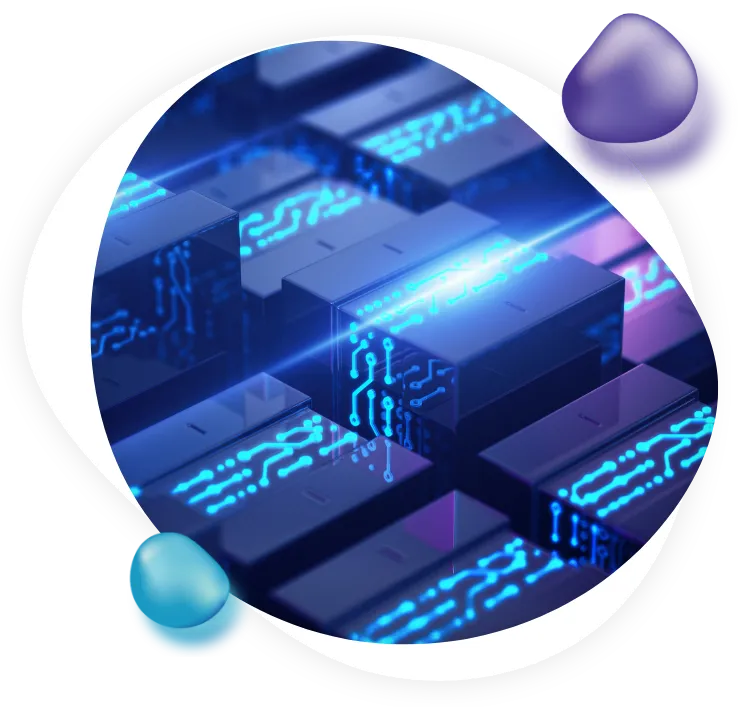
Built-in
best practices
We’ve fielded a lot of surveys. We want to share what we’ve learned over the years, so we’ve automatically included in the survey building process best practices for things like the number of questions, character limits, answer limits, and more. Have your own requirements? We won’t hold you back.
After you’ve designed your survey, our real-time automated insights dashboard will help you truly satisfy your curiosity.