We don’t tend to think of randomness as very useful in business. Generally, organizations avoid random things or consider the risks they need to manage. However, in the right context, doing something randomly can be the most effective way to achieve your objective—in the proper context, that is.
These days, data is quickly becoming an essential commodity. Knowing what consumers are asking for when they ask for it can be the difference between winning or losing in this competitive environment. Today, we’ll talk about probability sampling and how it can be a valuable methodology for acquiring data.

What is probability sampling?
To answer this question, we need to take a step back and first explain sampling. In research, the population you’re studying is typically too large to ask every member your questions. Sampling is the process of identifying a representative subset or sample of that larger population. Using this data (if the sampling is done correctly) we could infer conclusions about the larger population.
For example, when we see political polls on TV, they’ll usually show you the sample size in the fine print at the bottom of the screen, indicating that the statistics are based on a certain number of people within the larger population (i.e., 1,000 respondents between the ages of 18 and 35).
Now, what’s probability sampling? Probability sampling seeks to eliminate sampling bias by sampling based on completely random chance. Sampling bias occurs when certain population members are more likely to be selected. This could be due to many factors but can be removed from the equation by relying on probability theory.
This fancy-sounding term describes the same thing people have been doing for thousands of years—pulling names out of hats or drawing straws. However, nowadays, computers are used to ensure that sampling is done randomly to ensure that every member of the population has an equal chance of getting selected. By eliminating sampling bias, you ensure that your sample represents the overall population. This increases the quality of your data and makes it more usable for decision-making.
The difference between a sample and a population?
Population refers to the entire group you want to collect data on. These are the people you’re interested in learning more about. In the context of market research, the population is your target market. Your target market might be any group of people based on various factors (age, geographic location, income, etc.). That would be your overall population.
But it’d be far too expensive and difficult to hand a survey out to anyone who might fall within your target population. That’s where a sample comes in. The sample is the specific group of individuals you’ll collect data from. In theory, the larger the sample size, the more accurate your data will be because you speak to more population members.
On the other hand, the costs and amount of time to complete the survey increases the larger the survey becomes. This is why finding the perfect balance is key. An example of a population could be all college graduates in the United States. In contrast, an example of a sample could be 1,000 recent college graduates from various states who have agreed to respond to your survey.
Once you’ve identified your target population, you’ll need to use a systematic method of sampling this population. If you don’t rely on a system, you could introduce bias into the data, reducing its quality. We have already covered one method, probability sampling, but how does this compare to another sampling method—non-probability sampling?
Probability Sampling vs. non-probability sampling
Non-probability is another term for sampling methods. However, non-probability sampling refers to processes that are non-random in their sampling. Sometimes, non-probability sampling is a valid and appropriate way to collect data. However, whatever data you get from non-probability sampled research cannot be generalized to the larger population. Non-probability is often a fast and affordable way to quickly get qualitative results that can help you learn more about the population.
Generally, probability sampling is preferred because the data is quantitative and of higher quality. However, it’s essential to choose the sampling method that works best for you. It’s vital to be clear when presenting data whether or not the sampling method used was probability or non-probability. Although non-probability is often more affordable and faster than probability sampling, advanced tech can make running survey programs and sending questionnaires using random sampling pretty straightforward—regardless of the population size.
Types of probability sampling
Once you’ve decided to go with probability sampling, you’ll need to know about the different types. There are many techniques, but there are generally four well-recognized sampling methods:
#1 Systematic sampling
In systematic sampling, every member of a given population is assigned a specific number. Then, these numbers are selected based on a given interval and starting point. For example, if you were to start at 2, and then select every 3rd number (2, 5, 8, etc.), you would be using systematic sampling. Systematic sampling is recommended when there is a low risk of data manipulation.
Data manipulation occurs when a researcher changes the order of the data at one point or another. In this case, various factors could lead the researcher to order the data. For example, they might have organized the data by the age of the population members. In this case, the data would be manipulated, bias is possible, and systematic sampling is not advised. However, as long as the data is not likely to be manipulated, systematic sampling can be an effective way to get a truly representative sample.
#2 Simple random sampling
With the simple random sampling method, the subset of the population is randomly selected using a computer program. In this case, you would also assign each member of the population a number, just as you did with systematic sampling. However, the numbers are selected using a random number generator instead of choosing your samples based on intervals.
#3 Stratified sampling
In stratified sampling, the larger population (or target market) is divided into groups with similar characteristics. Random samples are chosen from each group once the population has been broken down into these subcategories. The results from each of these samples can then be put back together to get results that are representative of the overall population.
#4 Cluster sampling
Cluster sampling is similar to stratified sampling because both follow divide and conquer principles. However, in cluster sampling, the groups are selected randomly, and 100% of the members of those smaller groups are sampled for the study.
The simple way to do probability sampling
Regardless of the type of probability or non-probability sampling you want to use for your survey or questionnaire, aytm can help facilitate, or even run your next study. Our all-in-one platform combines a powerful sample engine with an intuitive survey authoring—complete with all the tools you need to analyze your data. If that sounds like a tool you’re interested in, go ahead and sign up now!
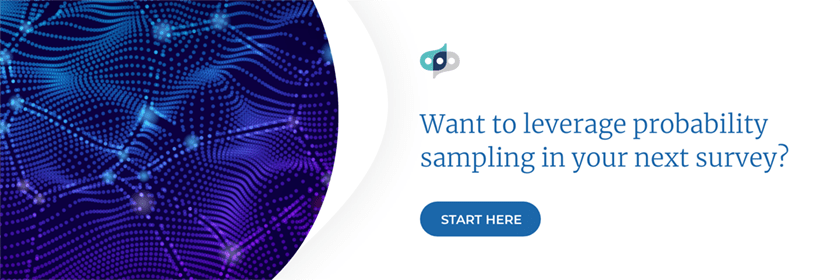