When developing a new product, you will complete several rounds of research to generate new ideas, optimize concepts, and position the final product in the marketplace. During the product development process, you’ll need to understand what features are most valuable or motivating to consumers. Two commonly used methods to quantitatively estimate the value of a product’s features include Conjoint Analysis and Discrete Choice Modeling.
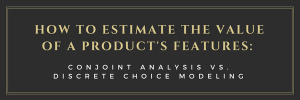
By gathering respondent rating or ranking data with a Conjoint approach, you are usually trying to understand which features are most important and which combinations, or specific bundles, of those features will yield the best product. Discrete Choice Modeling collects preference data from respondents making choices from sets of full propositions. While Conjoint Analysis and Discrete Choice Modeling are similar and both involve more sophisticated analysis, understanding the benefits and trade-offs of each will help you decide which methodology is best for accomplishing your objectives.
Full-Profile Conjoint Analysis
Full-Profile Conjoint Analysis looks at trade-offs consumers make when choosing between product attributes (such as brand name, speed, size, weight, price, color, etc.), and it calculates the attribute’s relative importance in choice. In a survey, respondents’ provide importance or purchase likelihood ratings/rankings on the various attributes. Each attribute can be evaluated on several “levels”.
For example, if “brand” is one of the attributes, brand may be evaluated on three different levels – meaning respondents would rate three different brands on the same scale. A preference scale value, or utility, is then created for each attribute level. A larger range among an attribute’s utilities suggests that, overall, the attribute has a high level of relative importance in choice. Additionally, the attribute level with the highest utility has greater importance. A small spread of utility scores indicates low importance for that attribute. Because the total utility for any bundle of attributes can be calculated using the component utilities, Conjoint data results in several possible “what if” simulations/scenarios.
Conjoint Analysis has many practical applications including optimizing product design, measuring the relative importance of features or attributes for individual consumers or segments of consumers, predicting choices and market shares, and quantifying the trade-off structure of preferences for all possible combinations of the product’s features. For best-practice, limit the number of tested attributes to around six, and test the attributes that you already know/suspect are most likely to drive purchase, so the data is useful and applicable. Also, be careful to create a balanced design where each attribute has a similar number of levels.
As with any methodology, it’s important to understand the trade-offs. Traditional Conjoint assumes that the whole equals the sum of the parts, so that any combination of the utility values can be added up to create a total utility. However, this may result in a product that doesn’t make sense or isn’t feasible, such as a grape lollipop that is the color orange. Using an “interaction variable” that combines two features into a single level, such as flavor and color, may be appropriate, but it does complicate design. Additionally, be aware that levels of attributes are not flexible: e.g., the same price range must be used regardless of brands being tested.
Discrete Choice Modeling
While a Full-Profile Conjoint approach typically presents each product attribute one at a time with respondents rating each one individually, usually on a purchase intention scale, Discrete Choice Modeling (sometimes referred to as Choice Based Conjoint) presents respondents with multiple sets of product attributes at once and respondents pick the set they prefer, or none of the sets.
So, consumers are providing a choice or preference as their response, which could be considered a more realistic task comparable to actual shopping behavior. In Discrete Choice Modeling, attributes and levels (like price) can be brand-specific and the interactions can be modeled, typically by aggregating the choices made by all respondents (unlike Conjoint where each respondent may be analyzed separately). Additionally, complex analysis using a multinomial logit procedure can be executed. Discrete Choice output is usually a share simulator. In a survey, respondents are presented with several choice scenarios – around 10 to 12 – that include varying brands and other attributes. As a researcher, you’ll design the sets so that each attribute level is equally likely to occur with each level of every other attribute.
Discrete Choice Modeling is the preferred approach when understanding pricing is the primary research objective since attributes can vary by brand and up to around 20 brands can be tested if needed. However, if you need to understand tradeoffs between attributes or need help optimizing the total product design, Conjoint data may be more useful.
The Takeaway
Full-Profile Conjoint Analysis and Discrete Choice Modeling are both excellent approaches you can utilize for product optimization research. Through Conjoint, you will learn which product features are most important by presenting respondents with each attribute individually and having them provide rating or ranking responses for each. Discrete Choice Modeling, on the other hand, collects preference data from respondents who make choices among “full products” (multiple attributes shown at once). Understanding the different capabilities of each will aid you in selecting the best methodology for achieving your research and business objectives.