We recently hosted a webinar on MaxDiff research called “Mastering MaxDiff: Best practice for optimal decision insights” During the webinar, we discussed best practices for designing MaxDiff studies, extracting meaningful data, and interpreting results to drive strategic decision-making.
What is Maximum Difference Scaling (MaxDiff)?
MaxDiff is an analytical technique used in market research to measure preference and importance among consumers. The goal of a MaxDiff is to help researchers understand which items to prioritize amongst a set by asking respondents to make trade-offs between the items—essentially asking them to indicate the “best” and “worst” options out of a given set, which subsequently quantifies the preference or importance of the items on a list.
From the MaxDiff exercise, utility scores are calculated and those get transformed into other metrics, like preference likelihood. These metrics not only give researchers an understanding of preference order, but how preferred certain items are to each other.
Here are some examples of testing where MaxDiff is ideal:
- Concept and idea testing
- Package and logo testing
- Claims and message testing
How does MaxDiff work?
Instead of a MaxDiff being a single question type that asks respondents to rank items, it breaks up the evaluation items into a series of smaller comparisons. It takes a list of your items to be compared, and shows them in a balanced order to each respondent 3, 4, or 5 at a time.
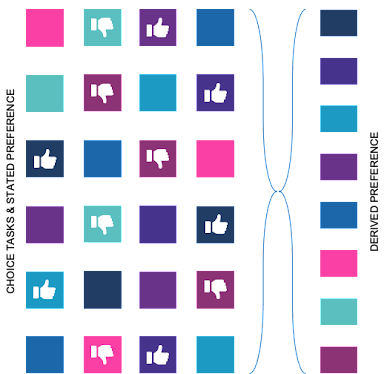
When should I use MaxDiff over basic ranking?
The MaxDiff question type is recommended for comparing more than 6 items, and can support up to hundreds of items. Basic ranking isn’t recommended for any more than 10 items. MaxDiff helps you understand the distance between the ranking of items. With ranking, you can determine whether one item is better than another but not by how much. MaxDiff allows you to dig in and understand and quantify the gaps between those items, to understand how much more preferred one item is to another.
Best practices for designing a MaxDiff exercise
When designing a MaxDiff, there are a few best practices to follow to ensure reliability of your results. These include:
Maintain consistency across comparison items
Maintaining a consistent level across all items is crucial. For instance, if you’re evaluating features of a car, compare elements like style against each other instead of mixing categories such as style vs. color. Within a category, like color, ensure that the items represent distinct choices, such as different colors but not contrasting a red car against a sedan.
Insure alternatives are testably different
When asking participants to make trade-offs based on their preferences, it's important that these alternatives are “testably different.” Each item should be noticeably different from one another. For example, when comparing modes of transportation, provide a variety of options such as car, train and bus. However, it’s important to not overrepresent a category comparing things like red bus, yellow bus, blue bus to car, train, etc.
Allow tech to bring consistency
Employ technology and specialized software to craft the underlying design of the MaxDiff. This ensures you don’t repeatedly present the same subsets of items, which can skew results. A balanced design is critical and an unbalanced one can compromise your outcome.
Create a positive respondent experience
Lastly, before launching your MaxDiff study, make sure that you’ve crafted a positive survey experience. Plan to ask enough choice tasks to gather at least two evaluations per item, but be mindful of respondent fatigue. It’s recommended to resent no more than 20 screens during a particular study. Like any other survey, conduct a thorough test to ensure the overall experience is positive. This will help to identify and correct potential issues in advance, leading to more accurate data collection and insights.
MaxDiff summary metrics
Below are two standard metrics used to analyze MaxDiff: preference likelihood, and utility scores. It’s important to note that no metric is not better than the other—the metric used should be determined by what is most important to a particular project.
- Preference Likelihood: The probability that a given item would be selected within the survey. The baseline percentage is determined by the number of items per screen programmed in the MaxDiff.
- Raw output from analysis that represent preference
- Basis for other, more commonly reported metrics with simpler interpretations
- Best choice for significance testing
- Utility Scores: Raw scores (centered around zero) that reflect directional preferences of items. Zero represents the average performance; the more positive an item's utility, the more it is preferred by respondents, and the more negative an item's utility, the less it is preferred.
- Likelihood of an item being chosen as most preferred over item(s)
- Can be rooted in different choice scenarios (e.g., best of 2, best of 4)
- Commonly reported as it is easily understood, highly interpretable
Advanced MaxDiff at aytm
aytm’s Advanced MaxDiff lets users test up to 200 items, but avoids overburdening respondents by only asking them to evaluate 3–5 items at a time. It comes in two varieties: Aggregate (formerly MaxDiff Express) and HB.
Aggregate focuses on collecting general aggregate information, without the intention of obtaining individual-level estimates. Typically, respondents would see 3–5 screens. And while it’s still possible to see results for a subset of respondents, keep in mind that the model only covers the subset of respondents—it doesn’t take into account the rest of responses.
In HB mode, the method focuses on collecting high-resolution individual-level data that can be analyzed by the Hierarchical Bayesian model. In typical settings, respondents would see 10-20 screens. Besides the additional option to extract individual logistic coefficients, HB mode makes it possible to look at analysis done on a subset of respondents with the confidence that the results are more robust—because the model allows for individual-level estimation.
We also recommend including a short instruction for the test so respondents understand the task and how many times they’ll be asked to perform it. For example:
- Reorder: Please rank the following items in the order of your preference: from most preferred on top to least preferred on the bottom.
- Best/Worst: Please select your best choice (thumbs up) and your worst choice (thumbs down).
- Image Grid: Please select your most preferred then least preferred image below
One more thing to note—sample size plays an important role in the success of a MaxDiff. And because it can be sensitive to the number of completes, we recommend a minimum of 400 per survey.
Get MaxDiff certified
Level up your expertise on MaxDiff research tests with our new MaxDiff certification for researchers. This certification is one of a kind in the industry, offering a completely free, comprehensive overview of this extremely popular research test.
This module will be followed by a partnering assessment to earn your aytm MaxDiff badge.
After the certification, you’ll be able to:
- Describe how a MaxDiff research test functions.
- Determine when to use a MaxDiff research test.
- Identify research needs that can be appropriately addressed by a MaxDiff research test.
- Demonstrate how to set up a MaxDiff on aytm's platform.
- Describe the difference between aggregate and HB modes of the MaxDiff research test.
- Demonstrate how to interpret the results from a MaxDiff research test.
GET CERTIFIED FOR FREE
Thanks for reading!
We just went through a brief recap of some key points discussed in our latest webinar on mastering MaxDiff. For a deeper dive into these concepts, including additional insights from our experts, be sure to check out the full recording of the webinar.
