Key Driver analysis is a common and relatively simple analytic tool for market researchers that can illuminate a number of actionable insights. Unlike advanced analytic tools like MaxDiff, conjoint, and segmentation, a key driver analysis can provide the earmarks of predictive analytics in a statistical analysis you can even run in Excel (using the Data Analysis ToolPak).
In order to conduct a successful and meaningful analysis, though, the power lies not so much in the regression analysis but rather in the design of the study. The questions that are asked and the manner in which they are asked are going to be the real indicators of how much predictive insight your key driver analysis can give you.
What is a key driver analysis?
A key driver analysis asks the question "Which of these elements is driving, or primarily responsible for, the outcome of interest?" Purchase intent or customer loyalty are common outcomes of interest in a key driver analysis, and the actionable insight is understanding which levers to pull to increase these.
Let's take the example of purchase intent. There are a number of factors that could cause someone to decide they are interested in purchasing your product: price, availability, appeal, effectiveness, and brand reputation (to name a few). If you want to increase consumers' purchase intent for your product, which of these areas should you focus on improving? In other words, which of these five factors drives purchase intent.
One way you could try to answer this question, without doing a key driver analysis, would be to explore each factor individually. You could run a pricing analysis to see which price increases purchase intent the most. You could do A/B testing to discover which version of your product has the most appeal. However, an important disclaimer from psychometrics will tell you that these features are not independent. Appeal will likely be influenced by effectiveness and brand reputation. The impression of an acceptable price will likely be affected by all of these factors simultaneously. How, then, do you parse them to discover the most effective lever to pull?
Regression analysis
Regression analysis, specifically multiple regression analysis, is the primary analytic method used for a key driver analysis. The statistical method lets you identify multiple inputs (e.g., price, availability, appeal, etc.) and provides their relative influence over the output (e.g., purchase intent). The power of the regression lies in this ability to evaluate multiple input features at the same time.
If the influence of product appeal can be completely explained by product effectiveness (people find it appealing BECAUSE it's effective, and for no other reason), then it's redundant to say both appeal and effectiveness drive purchase intent. It's just the effectiveness. A regression analysis is able to make these considerations and identify which features in the set are really the important ones.
In this way, a key driver analysis can really help to identify the most efficient places to focus efforts for improving your desired outcome. Rather than spending resources on trying to improve all features, you instead can focus on just one or two in earnest.
Survey design for Key Driver Analysis
There are a few important things to consider when designing your questionnaire for a key driver analysis.
First: The inputs and output
Start by clearly identifying what you want to explain—purchase intent, customer loyalty, even product appeal could be what you want to understand.
With this clear target in mind, then consider the different elements you believe could be influencing this. The key driver analysis can only account for those elements you measured. If you don't know that appeal and effectiveness are highly related, and you only ask about appeal, you will be unable to learn that effectiveness is really the star. On the other hand, if you already know that appeal is fully explained by effectiveness from previous research, don't overburden your analysis with elements you already know aren't all that important.
Finally, thinking about elements from an actionable point of view. If you learn that appeal is the biggest driver, does that give you a clear direction on how to improve purchase intent? Do you instinctively know how to improve the appeal? On the other hand, effectiveness has a clearer definition. You can more quickly identify steps to improve this. Likewise discovering that customer satisfaction drives customer loyalty doesn't really help you much if you don't know the steps you need to take to improve satisfaction.
Second: Response options
Statistical analysis, particularly regression analysis, is about understanding variance—how much individual answers change from person to person. Without variance, there is nothing to explain! The more variance available in the data, the better the regression analysis can identify patterns.
To allow for good variance in the data, questions intended for use in the regression analysis should have robust scales that allow for reasonable variation in responses. In psychometrics, a general rule of thumb is a scale of 7-points is the minimum size that can offer sufficient variation and be treated numerically (idea for regression analysis). Additional research has demonstrated that respondents find scales of 7–11 points are adequate for capturing the nuances of their opinions without being too granular. Of course, some elements may not be amenable to numeric scales of this size, but in the case of capturing attitudes and intentions these recommendations can lead to more accurate and insightful results.
Third: Respondent survey flow
It is not uncommon in market research questionnaires for respondents to only answer a subset of questions or be allowed to provide an answer option of "not applicable.” Unfortunately, in these cases, this data would not be usable for regression analysis. It is necessary for data to be complete to search for patterns between the variables. Although there are data cleaning methods that can account for missing data, these are not meant to be used when there is systematically missing data.
A good key driver questionnaire will:
- Have measures for clear, actionable features and the target outcome
- Measure attitudes/opinions on scales of sufficient length (e.g. 7–11 items)
- Ensure all respondents are able to provide an answer for every measure
With data captured in this way, using your favorite tool to conduct your regression analysis will provide you with reliable, actionable insights into how to improve your objectives.
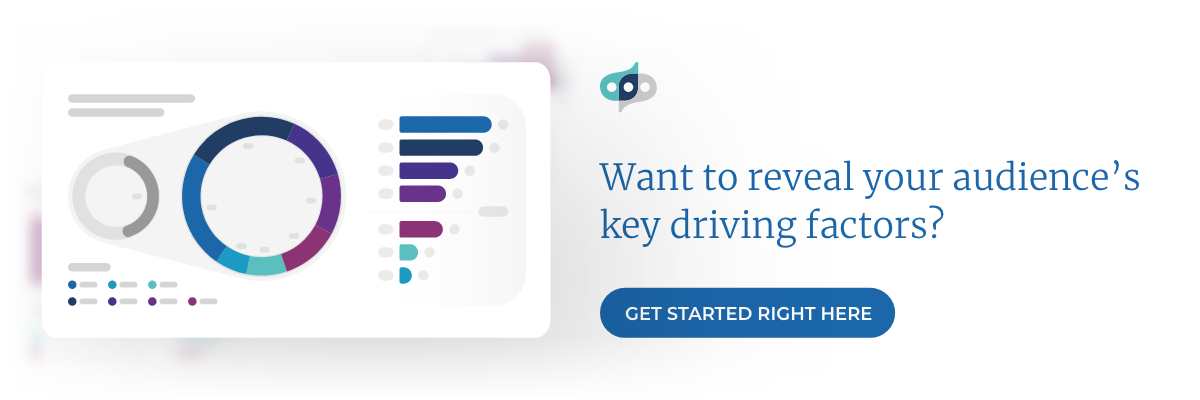