The disruptive potential of AI-driven research is already apparent across the industry. AI offers a promising future to enhance market research capabilities, unlocking insights at speeds and scales that were previously inaccessible to the average researcher. But it's important to recognize the risks these technologies pose, including over-automation, unverified findings, security vulnerabilities, and improper application if not strategically managed. Anyone integrating AI should take a nuanced approach to maximize value while proactively mitigating limitations. That starts with getting familiarized with the common terms of AI and understanding common implementations. So let’s take a closer look at the common buzzwords you’ll see in the field of machine learning and AI.
The market researcher’s AI glossary
AI is a rapidly evolving field, and with so many new buzzwords constantly emerging, it can be hard to separate fact from fiction and keep up with the latest developments. Understanding these terms and concepts can lay a powerful foundation that will empower you to make informed decisions within the realm of artificial intelligence—so let’s dive in.
Building block definitions
First, let’s set the stage by going over the different terms that form the basis of artificial intelligence as we know it. We’ll call these “building block” definitions.
- Algorithm: This is a set of instructions or rules that machines follow to solve a problem or accomplish a task.
- Big Data: These are datasets considered too large or complex to process using traditional methods. This term encompasses the analysis of massive sets of information to glean valuable insights and patterns that improve decision-making.
- Quantum Computing: Known as a cutting-edge approach, Quantum Computing leverages quantum bits (qubits) to perform certain types of calculations significantly faster than classical computers.
- Model: Models are when computers use math, data, and computer instructions to create representations of real-world events. They also can predict what’s happening—or what could happen.
- Computer Science: The discipline of Computer Science includes the study of algorithms and data structures, computer and network design, modeling data and information processes, and artificial intelligence.
Disciplines of Computer Science
Now that we have some of the basics down, let’s dive into the different disciplines of Computer Science to distinguish the differences between the various buzzwords we’ve been hearing about.
- Artificial Intelligence (AI): This term widely defines the simulation of human intelligence processes by machines programmed to think and learn like humans. The goal is to perform tasks that typically require human intelligence, such as visual perception, speech recognition, decision-making, and problem-solving.
- Data science: The science of extracting insights from data using scientific methods, algorithms, and systems. It encompasses a wide range of activities, including data collection, data visualization, and predictive modeling to solve problems.
- Cognitive Computing: This is a subset of AI that aims to mimic human cognitive abilities, such as learning, understanding language, reasoning, and problem-solving.
- Deep Learning: This specific subfield of machine learning uses neural networks to process data hierarchically and extract complex features. It is particularly effective in tasks like image and speech recognition.
- Machine Learning (ML): This is a subset of AI that allows computer systems to learn and improve from experience without being explicitly programmed. ML algorithms enable machines to recognize patterns, make predictions, and improve their performance over time.
Types of models
To understand the various types of AI, we need to look at the different kinds of models and the configurations that shape ways they can be leveraged.
- Bayesian Networks: These are statistical models that represent probabilistic relationships among a set of variables, used for reasoning under uncertainty with incomplete information.
- GPT (Generative Pre-trained Transformer): This is a family of large-scale language models known for their ability to generate human-like text. GPT-3 and GPT-4 are some of the most known versions, developed by OpenAI.
- Large Language Model (LLM): These machine learning models are trained on huge amounts of data to understand and generate human-like language. Large refers to the use of extensive parameters by language models. For example, GPT-3 has 175 billion parameters, making it one of the most significant language models available at its time of creation.
- Neural Network: These models are inspired by the human brain’s structure and function. It consists of interconnected nodes (like neurons) organized into layers to process and transform data and make predictions based on input.
Applications of models and AI
Now that we understand the basic structures of these different types of models, let’s take a look at the different ways this technology is put into practice.
- Computer Vision: This is a field of AI that enables machines to interpret and understand visual information from the world, such as images and videos.
- Chatbot: These are computer programs that use Natural Language Processing (NLP) and AI to simulate human-like conversations with users, typically deployed in customer support, virtual assistants, and messaging applications. This isn’t necessarily powered by AI.
- Natural Language Processing (NLP):This is a subfield of AI focused on helping computers to understand the way that humans write and speak, often used to analyze and generate text.
- Sentiment Analysis: This refers to the process of using NLP techniques to determine the sentiment or emotion expressed in a piece of text, often used in social media monitoring and customer feedback analysis.
Evaluating AI
Before we finish, allow us to share some insight into the different parameters we use to evaluate AI, and the importance of understanding some of the challenges you may encounter.
- Bias in AI: AI bias refers to the tendency of a model to make certain predictions more often than others. Bias can be caused due to the training data of a model or design decisions.
- Bias mitigation: These are techniques and strategies used to reduce or eliminate bias in AI systems, ensuring fairness and equitable outcomes.
- Consent and AI: Be aware that systems that collect, process, and generate personal and proprietary data can intensify ongoing concerns around consent, such as offering adequate notice, choice, and options to withdraw from sharing data.
- Ethics in AI: It’s critical to take into consideration moral principles and guidelines when developing and deploying AI systems. This can help ensure new forms of technology are used responsibly and do not harm individuals or society.
- Explainable AI (XAI): The concept of designing AI systems that can provide transparent explanations for their decisions, enables humans to understand the reasoning behind AI-generated outcomes.
- Hallucination: AI hallucination refers to the instances where a model produces factually incorrect, irrelevant, or nonsensical results due to lack of context, limitations in training data, or architecture.
- Privacy and security in AI: Keep in mind that censoring or restricting certain data sets can help protect individuals' privacy, security interests, and proprietary information.
Wrapping up, remember that the newest advances in AI and ML have been achieved thanks to powerful computers, massive datasets, and extremely large models with billions of parameters. Notably, these core ideas were developed in the late 20th century, so they aren’t new in theory, just in execution. Now, notions that were theoretical are achievable at scale.
Capabilities and applications of AI in consumer insights
As outlined previously, the landscape of AI underwent a significant transformation in late 2022 and early 2023 with the introduction of generative AI tools for consumers. While experts had been intrigued by generative AI since the debut of GPT-2 in 2019, it is only recently that its groundbreaking potential has become evident to businesses.
So, what can insights professionals expect in this new era?
- Rapid processing of qualitative data
- Predictive analytics
- Image and video analysis
- Consumer segmentation
- Survey generation and analysis
- Automated report writing
Seek a measured approach in order to build a tech-enabled future
When it comes to AI, sustainable results come from reality, not mythology. AI is not a magic wand that automatically solves problems like bias without diligent governance. But it also isn't to be feared as an uncontrollable force that will replace human judgment.
The measured path is to carefully pilot applications that augment human capabilities and insights—to establish thoughtful oversight and validate against research ethics principles. We should embrace AI as a versatile set of tools that, with responsible design, can drive business value while advancing societal good.
And while we’re excited about the possibilities these new technologies present, our approach requires rigorous testing in controlled environments, iterating on our hypotheses, and only bringing solutions to market that pass our rigorous development process. We’re continuously exploring, and experimentation is an integral part of our DNA.
To that end, aytm remains committed to delivering quality products and experiences for insights seekers today while building a tech-enabled future for the curious of tomorrow. We also pledge to absolute transparency when it comes to how your data is or isn’t being used—empowering you to opt in or opt out of using any AI-powered features across the board.
As we move forward, we’ll continue to take this careful yet agile approach to AI, going beyond the buzzwords and setting pragmatic expectations. Optimism is good. But let’s ground it in reality. There’s no doubt that AI will transform market research, but it’s up to us to understand how.
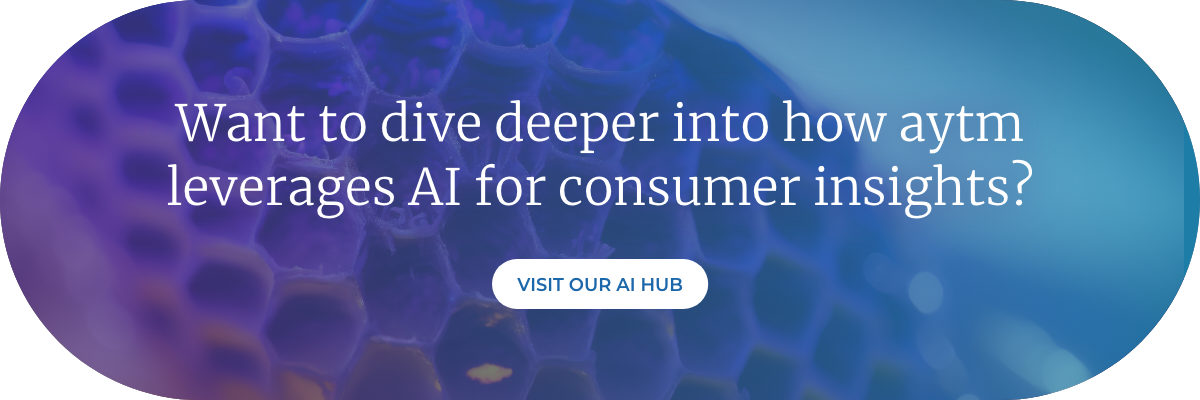