Unlike traditional product research focusing on individual offerings, line optimization delves into the collective strength of your product portfolio. This approach aims to answer critical questions such as which innovations can attract the most new consumers, how to strategically price your products for optimal trade-up, and which packaging changes can enhance your competitive edge. In this article, we'll explore three powerful tools employed in line optimization research: TURF analysis, conjoint, and shelf testing. Each one offers unique insights into consumer behavior and market dynamics, empowering you to make informed decisions that drive growth and success. So whether you're a seasoned researcher or new to the field, understanding these methodologies can revolutionize your approach to product strategy and market positioning.
Line optimization
Let’s start with the basics: What is line optimization research, exactly? When we talk about line optimization, we can be referring to any research that informs the setup of or changes to a product portfolio. Distinct from product research, where we may use similar methods to maximize the appeal of a specific product or offering, line optimization research focuses on maximizing the strength of a collective group of products working together under a brand/subline structure.
Line optimization research seeks to answer questions like:
- Which of my potential new innovations bring the most new users to my brand portfolio?
- How do I arrange pricing across my three tiers (good, better, best) to maximize trade-up?
- Which package changes allow me to capture the most competitive share of the market?
Often this research is done in a competitive context (though not necessarily) so that researchers can evaluate the results in real terms of consumer conquesting, cannibalization, alienation, etc.
Comparing methodologies
When conducting quantitative line optimization research, aytm employs three powerful tools: TURF analysis, line optimization choice-based conjoint (or CBC), and shelf testing. There are benefits and drawbacks to each approach, which should be considered when determining which is right for your objectives.
A loose framework for thinking about how these three approaches relate would be through the lenses of cost, complexity, and “realism.”
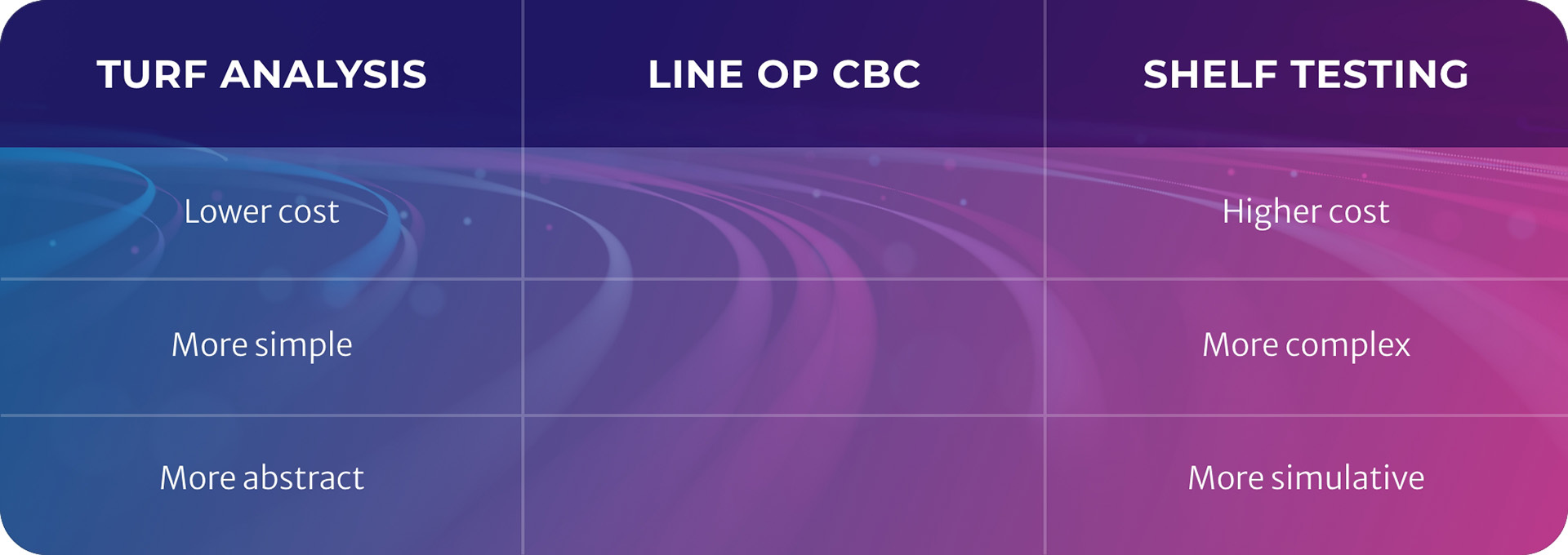
Evaluating each approach on these factors helps identify their relative strengths and weaknesses.
TURF analysis
Commonly used in line optimization research, TURF (Total Unduplicated Reach and Frequency) involves assessing products’ purchase intent at the respondent level, and then compiling that information to understand which products appeal to which consumers. With this respondent-level data, we can see which products “reach” the most consumers (that is, which products have positive PI among the most consumers). More importantly for the purpose of line optimization research, we can see the overlap in “reach” between products.
Here’s a high-level hypothetical to illustrate why this is important: If your brand has one existing product in market and the goal is to launch one new innovation this year (and only one), your goal is often to grow the brand’s overall market penetration, and that means reaching new consumers. Therefore, you need to identify the innovation that has the least overlap with your existing product, or in other words, the innovation that provides the most incremental growth to your existing line.
Analysis of this overlap is conducted through a TURF Simulator, that allows the researcher to “add” products to a hypothetical scenario and see that total unduplicated reach (the total reach of included products minus any overlap) for various combinations of products.
PROS
Some of the advantages of TURF include the fact that this analysis is based in readily-understood terms (purchase intent). TURF analysis can also be lower cost and easier to execute due to its simplicity.
CONS
The disadvantages are that this analysis is based on purchase intent, which some feel can be overstated. Also, while you can test competitor benchmarks for their PI, TURF lacks any way of showing a researcher where interest in their product goes or comes from between each scenario. In other words, it cannot address questions of competitive sourcing/capture or cannibalization.
Line optimization conjoint
You may be familiar with typical product-based conjoint, where the individual features of potential products are assessed to create the best overall product package. Line optimization conjoint modeling works similarly, but it takes things up a notch strategically; instead of revealing the optimal product from a set of variable attributes, it determines the optimal portfolio from among a set of variable products.
To do this, the inputs to the conjoint are represented as whole SKUs (not product features), and that list of SKUs will include all existing products—relevant competitor products, and any proposed changes (whether those be new innovation SKUs, existing products at a different price, existing products with a different artwork, etc). Respondents then complete the conjoint exercise, where they see 3–4 of these SKUs (with pricing) and a “none” option and are asked to indicate which they would be most likely to buy. They repeat this task a number of times as the conjoint shuffles the various products around. The conjoint then develops utility scores from the results which show how preferential each product is.
Like TURF, the key analytical tool is a simulator, which allows researchers to “create” product lines from any of the tested SKUs and measure the overall purchase share for their brands/sublines. Importantly, the conjoint approach is done in a competitive context, and that allows researchers to know how they can affect competitor share with changes to their own line up. And, similarly, line optimization conjoint allows the researcher to see any cannibalization within their own lines.
PROS
Advantages of CBC include the fact that it takes the TURF’s read on incrementality up a notch, and adds the ability to see cannibalization and sourcing information. It also allows for optimization of larger lines and a larger number of proposed line changes and scenarios.
CONS
The disadvantages of a CBC are that they’re usually higher cost than TURF due to increased sample requirements (they’re also sometimes more difficult to explain). To that end, CBC involves more service support through simulator build and interpretation—so they may be less DIY-friendly.
Shelf testing
Shelf testing is a powerful tool for conducting line optimization. To explore various line up scenarios, the researcher will build a virtual product shelf which includes the proposed lineup as well as relevant competitors. Respondents are asked to shop the shelf as they normally would. It’s as straightforward as that!
Once the study is out of field, the researcher can then compare the total share of purchase selections their brand captured under each scenario, and can even compare that to competitive shares to see any important interactions.
PROS
An advantage to this is that the entire research is built in a real-world shopping simulation, which many researchers prefer as it grounds the results in easy-to-understand findings. It also shows a comprehensive view of how share is affected under each scenario—brands, sub brands, and competitors—everything can be tracked and understood.
CONS
The downside is that each possible scenario or lineup change needs to be built into a separate shelf. This means that the scenarios must be defined up front and there’s no room for exploring additional options once the study fields. Compare that with CBC, where as long as the SKUs in question were tested, you can use the simulator to see any possible combination or lineup that comes up further down the road. Also, since each scenario requires its own shelf, this method can be more costly in terms of setup and sample requirements, and is limited in the number of scenarios that can reasonably be tested (somewhere from 5–8)
Closing thoughts
Wrapping things up here, line optimization research offers invaluable insights into maximizing the effectiveness of your product portfolio. By leveraging methodologies such as TURF analysis, choice-based conjoint (CBC), and shelf testing, you can uncover hidden opportunities for growth, refine your pricing strategy, and outmaneuver competitors in the market. So whether you're a brand manager, product developer, or market researcher, embracing these approaches can lead to more informed decision-making and ultimately, greater success in today's competitive landscape.
Here’s how aytm can help
If you’re in the thick of line optimization, don’t feel like you have to take it on your own. The experts at aytm are always standing by to help—whether it’s helping you set up your first studies, or taking over the project completely. And if you feel near-confident enough to tackle it yourself, you can always head over to the Lighthouse Academy to find helpful articles, tutorials and instructional videos of how to make it happen within the aytm platform!
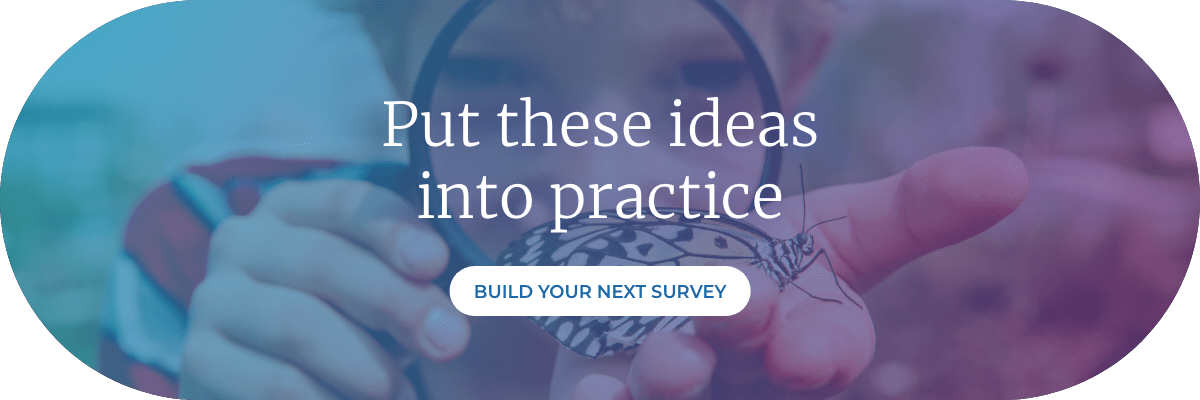