There’s an old expression most of us have heard about not being able to see the forest for the trees, meaning that one is looking so carefully at the details that they can’t see the big picture — sound familiar?
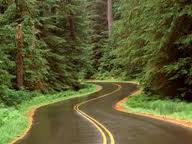
Think about it like an artist. To paint a picture of a forest, she must paint each tree, but only by stepping back once in a while can she see what her finished painting will become. Well, it’s a decent metaphor for data analysis, too. It’s tempting to zero in on all the juicy details and forget, in our excitement, to step back and appreciate the big picture our data is painting. Many researchers, especially newer researchers, get so excited about the many cool things we can do with data analysis that they forget the importance of the big picture. As you’ve discovered using the AYTM platform, it’s easy to see how answers to your questions vary by income level and gender and such; but focusing too soon on those variations can also distract you from the forest — the universal results.
What’s Universal?
• The ForestBefore you jump into sub-group analysis, take a step back and look at what’s universal. Did 80 percent of your respondents have a specific attitude or preference? If 80 or 90 percent of your target market prefers a specific color or feature, or all want to purchase your product through a particular retail outlet, that’s pretty powerful stuff. • Not the TreesThe fact that those results also varied 10% by gender is interesting, but probably not particularly actionable. It’s more of a curiosity. Ok, 70% of males preferred this color, and 90% of females did, but are you going to change your recommendations when on average 80% liked that color? Not likely.
First the Forest, Then the Trees
We begin our analysis with the forest first—the universal results—but we don’t skip over the finer sub-group analysis. Once you’ve gotten a general picture of the results, it’s time to begin looking closer to find those bits of wisdom that flesh out the picture we’re painting. Sub group analysis provides detail and character to our results. Further, once we get to that level of analysis, we often find that certain types of sub-group analysis will be more interesting than others. In some product categories, the most interesting results may are by gender. In others, by income level or education. As you do more research, you will learn what types of customer attributes reveal the most useful results.
Differences change with time
Males and females have different behaviors. Older and younger consumers behave differently. Right? Maybe not.In some categories there are definite differences between men and women, but those differences are not the same today as they were in 1955 — or even 1995. Age is another factor that can make a difference, but if you look at certain consumer behaviors you’ll see that this isn’t absolute either. Online shopping used to be very oriented towards younger people, and older folks simply weren’t comfortable with it. Today, however, a lot of that age group difference has gone away as the more senior among us have gotten comfortable online. The lesson here is to keep current: Just because a sub-group analysis is powerful today doesn’t mean it will be tomorrow.
First things First
Bottom line, when you’re ready to begin analysis, start at the beginning. Before you look at sub-groups, do an initial read of your data by looking at the totals. Look for those results that represent your entire survey population, and look at the overall distributions. An artist starts with a pencil and sketches in the general lay of the scene before she paints a single tree, and it’s really no different in data analysis. Start general, get specific, and stay open to inspiration!